Abstract
We conduct large-scale studies on 'human attention' in Visual Question Answering (VQA) to understand where humans choose to look to answer questions about images. We design and test multiple game-inspired novel attention-annotation interfaces that require the subject to sharpen regions of a blurred image to answer a question. Thus, we introduce the VQA-HAT (Human ATtention) dataset. We evaluate attention maps generated by state-of-the-art VQA models against human attention both qualitatively (via visualizations) and quantitatively (via rank-order correlation). Overall, our experiments show that current attention models in VQA do not seem to be looking at the same regions as humans.

Bibtex
@inproceedings{vqahat, title={{Human Attention in Visual Question Answering: Do Humans and Deep Networks Look at the Same Regions?}}, author={Abhishek Das and Harsh Agrawal and C. Lawrence Zitnick and Devi Parikh and Dhruv Batra}, booktitle={Conference on Empirical Methods in Natural Language Processing (EMNLP)}, year={2016} }
VQA-HAT Dataset
Human attention image files are named as "qid
_n
.png".
qid
refers to question ID according to the VQA dataset (v1.0), and n
iterates over multiple attention maps per question.
For example, 1500070_1.png, 1500070_2.png, etc.
n = 1 for the training set and n = {1,2,3} for the validation set.
Download links are given below.
58,475 attention maps
4,122 attention maps
Human vs. Machine Attention
Machine-generated attention maps on COCO-val for Stacked Attention Network
(Yang et al., CVPR16), HieCoAtt (Lu et al., NIPS16) and Judd et al, ICCV09
are available for download here.
For SAN and HieCoAtt, image files are named as "qid
.png", and
for Judd et al., "coco_image_id
.jpg".
Examples of human attention (column 2) vs. machine-generated attention
(columns 3-5) with rank-correlation coefficients are shown below.
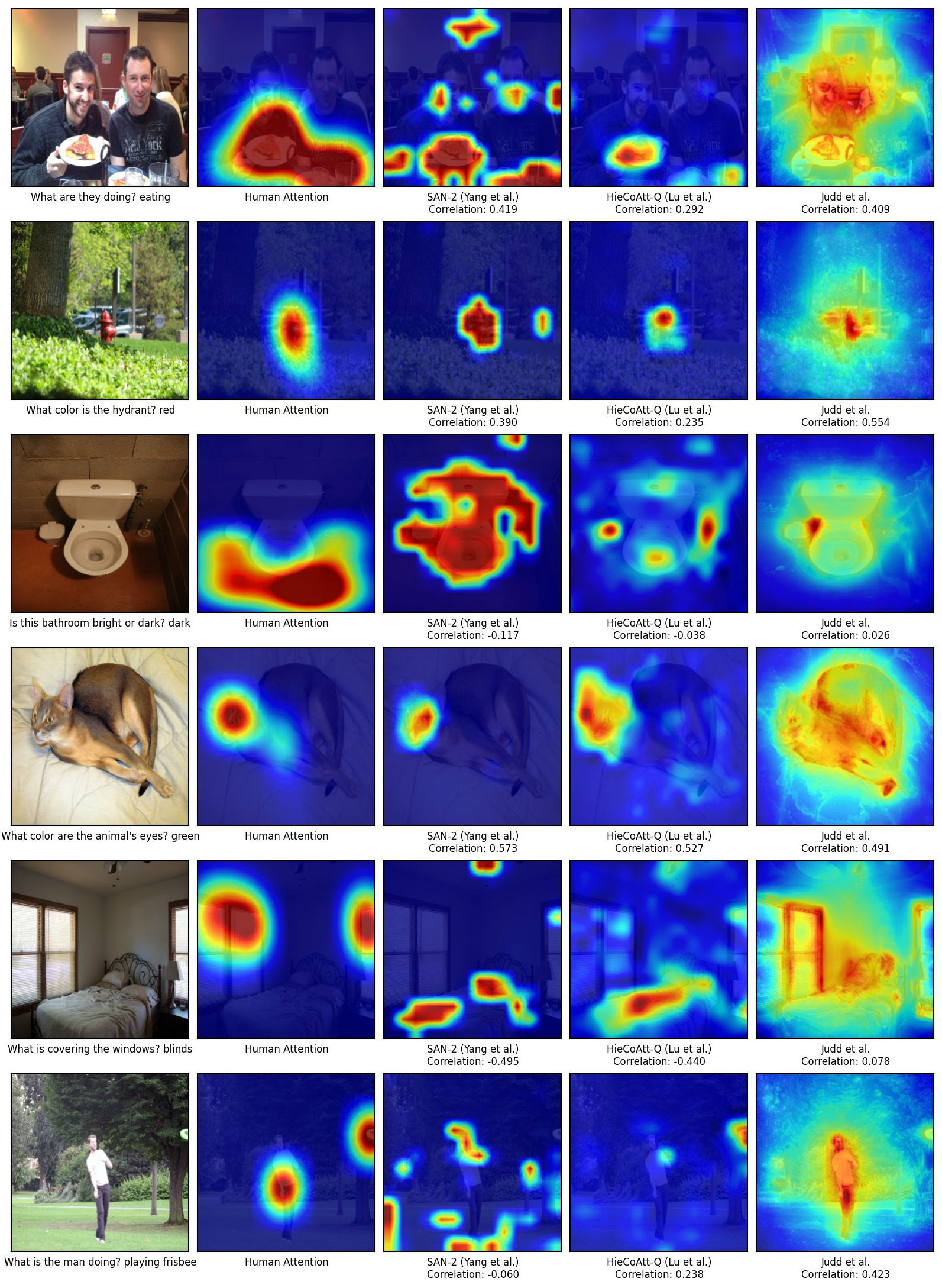